Advertising Ecosystem: What is the cost on user’s online privacy?
Introduction
We constantly leak personal data to various entities while browsing the web or generally interacting with web services. For example, such leaks happen when we use mobile apps or visit shopping and news websites, all of which may display advertisements to us. Interestingly, during our browsing, we are typically targeted with specific ads based on (a) the website visited (e.g., jacuzzi ad in a pool website), (b) the profile we have demonstrated (e.g., ads for expensive cars to people with high income), or (c) current purchases (e.g., ad for specific pair of shoes to people who showed interest in buying them). In all these cases, advertisers collect personal and behavioral data of users and use such data to target them accordingly, as well as share the data with other, 3rd party companies such as platforms for data management, trackers, credit score companies, etc.
To protect from, and inform online users of such practices, novel methods are needed to detect and stop the collection and sharing of user personal data. A team led by Nicolas Kourtellis, from the Scientific Group of Telefonica, has spearheaded a research effort in the last few years and under the funding of Telefonica I+D, and European Commission H2020 projects such as Concordia, to design, test and release tools that support web transparency and personal data leakage detection. These tools help users become aware of data leaks, measure how deep such leaks travel in the ad-ecosystem (i.e., how many 3rd parties learn about a user’s data from a single leak), and ultimately protect online users from further exposure.
YourAdvalue
One of these tools was designed[1] to analyze the ads shown to users while browsing the web and identify which of them were driven by programmatic instantaneous auctions (advertisers automatically bid at real-time how much they are willing to pay to show a specific ad to a given user at the given moment). Such ads have increasingly been used on the web, for both desktop and mobile devices, and cost 10s of billions to deliver. Given their growing dominance in the ad-ecosystem, and the association that ads have with users’ profiles, and how much they cost to be delivered, online users should know how much advertisers spend to target them, or in other words, how much the users are worth to the advertisers.
The proposed tool, called YourAdValue (Figure 1), was implemented and tested. It allows a user to calculate in real time the individual cost of the ads delivered to her, in CPM-Euros (cost per mille, i.e., cost for thousand ad impressions delivered). Using this tool, the team analyzed these costs and found that advertisers, based on users’ personal data, paid in a year just ∼25 CPM-Euros, and less than ∼100 CPM-Euros to 3/4 of the users. They also found that a small portion of very “expensive” users (∼2%) cost 10-100x more to the ad-ecosystem than the average user.
Furthermore, with a follow-up study[2], the team compared the costs on digital advertising for the advertiser and the user, in an attempt to identify how equal, or even comparable these costs are. Surprisingly, the results show that these costs are unbalanced, with the majority of users sustaining a significant loss of privacy, when the monetary cost they pay is, on average, 3 times more than what the advertisers are charged to deliver the given ads: the median advertiser paid 0.71 CPM-Euros, but the median user paid 2.2 CPM-Euros of their data-plan to download these ads! Moreover, advertisement and analytics-related traffic was 1/5 of users’ total traffic, and ~8.2% of the data-plan volume of an average mobile user. In fact, this traffic can potentially consume up to ~9% of a user’s phone power, just by considering the additional network overhead imposed!
CONRAD
Another tool, CONRAD[3], was designed to detect leakage of user private data through cookies and other ad-tracking mechanisms. As it is well known, the primary identification mechanism of users on the Web is through cookies, where each web entity assigns a userID on the user’s side (device). However, each tracker knows the same user with a different ID. So how can the collected data from entity A, be sold and merged with the associated user data of the buyer (entity B)? Cookie Synchronization facilitates that two or more entities merge the user data they own in the background, but also reconstruct the user browsing history, bypassing the same origin policy (Figure 2).
CONRAD is capable of detecting and exposing such synchronization transactions and also detect leakage of user personal data, leading to some interesting insights:
- Omnipresence: Almost all users (~97%) are exposed to cookie synchronization at least once in a year. In fact, the median user is synced at least once within the first week of browsing, and his userID gets leaked to 3.5 domains, on average.
- Big-player dominance: Three top ad-companies learn more than 30% of all userIDs, each.
- Impact on privacy: With cookie synchronization, web entities know ~6.75x more about an average user.
- Private data that can leak with cookie synchronization: name, gender, age, date of birth, physical/email address, location, username and password, phone number, etc.
These results indicate that online users experience high costs due to delivered ads: device battery and data-plan consumed due to downloaded bytes for ads, and loss of cyber-privacy due to private data leakages from cookie synchronization. All such costs significantly outweigh both the efficiency of the received ads, and the cost paid by the ad ecosystem to deliver them to the user’s device. Thus, it remains unclear whom the current advertising model benefits, apart from the ad-delivery and targeting companies.
EyeWnder
On the same line of work, in a new study[4], the team designed, implemented and tested EyeWnder, a novel advertisement auditing system, that uses crowdsourcing (i.e., asking the users to provide some input to the system for better tuning of the detection algorithm) to reveal in real time whether an ad shown to a user has been targeting them or not. Detecting such ads is difficult, with current methods being effective, but requiring the aggregation of personal data from users (such as browsing history and delivered ads) in centralized repositories for analysis and labeling. Even though crowdsourcing simplifies the detection of targeted advertising, it has the same requirement for reporting to a central repository the impressions seen by different users, thereby jeopardizing the user privacy. EyeWnder breaks this deadlock with a privacy preserving data sharing protocol that allows the tool to compute global statistics required to detect targeting, while keeping private the ads seen by individual users and their browsing history. In effect, EyeWnder offers the means (Figure 3) to end-users and data protection authorities to conduct independent audits and decide which ads are targeting sensitive categories of users, as well as for testing the credibility of ad-choices and related self-regulation initiatives.
Talon
Worryingly, and in search for new ways to optimize the effectiveness of delivered ads, advertisers have introduced new advanced paradigms such as cross-device tracking (CDT), to monitor users’ browsing on multiple devices and screens, and deliver (re)targeted ads in the most appropriate screen. Obviously, this practice has led to even greater privacy concerns for the end-user. To detect CDT in a systematic and repeatable fashion, the team proposed[5] a novel methodology and implemented it in a framework called Talon, which allows experimentation with multiple parallel devices, experimental setups and settings. This new methodology is based on emulating realistic browsing activity of end-users (i.e., personas) from different devices, and thus triggering and detecting CDT ads. Talon is capable of detecting such CDT ads with high average precision (up to 92%). With Talon, one can also show that even if the user (persona) is employing incognito browsing, CDT is still possible to be performed, and detected with up to 73% average precision.
Conclusion
One thing is certain: User data collection and sharing activities done without users’ explicit consent can be illegal with hefty penalties under recent EU regulation ( GDPR and ePrivacy). It is, therefore, important for the design, development and distribution of practical, web transparency tools such as YourAdValue, CONRAD, EyeWnder, and Talon, which are readily available to privacy researchers, regulators and end-users. Both tech-savvy and average users can utilize these tools to investigate personal data leakage and anonymity loss they experience while browsing, due to the ad-ecosystem’s obscure practices.
References
[1] P. Papadopoulos, N. Kourtellis, P. Rodriguez Rodriguez, N. Laoutaris. If you are not paying for it, you are the product: How much do advertisers pay to reach you?
Conference: ACM Internet Measurements Conference (IMC), London, UK, 2017.
DOI: https://doi.org/10.1145/3131365.3131397
Preprint: https://arxiv.org/pdf/1701.07058.pdf
[2] P. Papadopoulos, N. Kourtellis, E. P. Markatos. The Cost of Digital Advertisement: Comparing
User and Advertiser Views.
Conference: ACM Web Conference (WWW), Lyon, France, 2018.
DOI: https://doi.org/10.1145/3178876.3186060
Preprint: http://www.protasis.eu/m/filer_public/db/3e/db3ee56c-699f-4005-909c-392e1c2cdc32/www18_papadopoulos.pdf
[3] P. Papadopoulos, N. Kourtellis, E. P. Markatos. Cookie Synchronization: Everything You Always Wanted to Know But Were Afraid to Ask.
Conference: ACM Web Conference (WWW), San Francisco, USA, 2019.
DOI: https://doi.org/10.1145/3308558.3313542
Preprint: https://arxiv.org/pdf/1805.10505.pdf
[4] C. Iordanou, N. Kourtellis, J. M. Carrascosa, C. Soriente, R. Cuevas, N. Laoutaris. Beyond
content analysis: Detecting targeted ads via distributed counting.
Conference: ACM Conference on Next generation Networking, Orlando, USA, 2019.
DOI: https://doi.org/10.1145/3359989.3365428
Preprint: https://arxiv.org/pdf/1907.01862
[5] K. Solomos, P. Ilia, S. Ioannidis, N. Kourtellis. Talon: An Automated Framework for Cross-Device Tracking Detection.
Conference: USENIX RAID, Beijing, China, 2019
DOI:
Preprint: https://www.usenix.org/system/files/raid2019-solomos.pdf
Short Bio for the author:
Dr. Nicolas Kourtellis is a Research Scientist in the Telefonica R&D team, in Barcelona. He holds a Ph.D. in Computer Science and Engineering from the University of South Florida (2012). He has published more than 60 papers, and presented his work in top academic conferences and journals. His primary interests are 1) user online privacy and leakage of personal data, 2) characterization of online user behavior on social media, 3) streaming mining.
Figures
Figure 1: The YourAdValue tool in action. The interface informs the user how many ads were detected, how much these ads cost to the advertisers that sent them (two types of ads: with determined price and estimated price), how the user can enable/disable the tool, the reporting of data for further research, etc. More information on how to install and use it: here.
Figure 2: Example of two entities (advertiser.com and tracker.com) synchronizing their cookieIDs. Interestingly, and without having any code in website3, advertiser.com learns that: (i) cookieIDs userABC==user123 and (ii) userABC has just visited the particular website. Finally, both entities can conduct server-to-server user data merges.
Figure 3: EyeWnder allows the user to annotate a detected ad as targeting the user or not. To help the user with their decision, the tool offers various global statistics about the appearance of the specific ad in the browsers of other users, their demographics and geography.
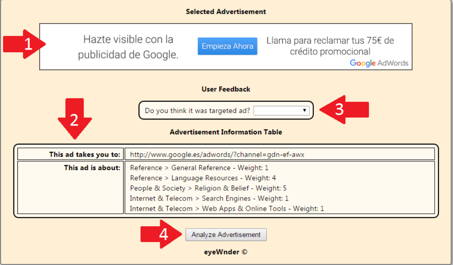
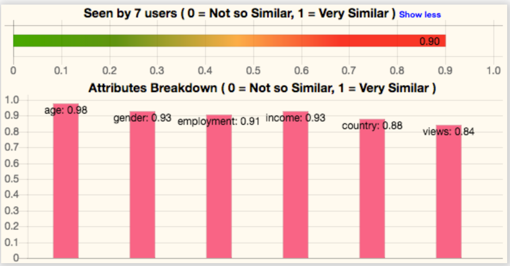
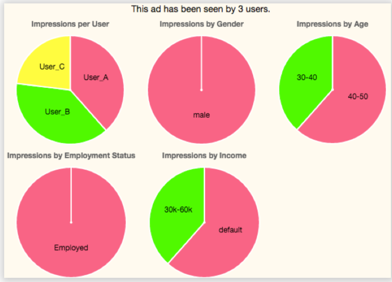
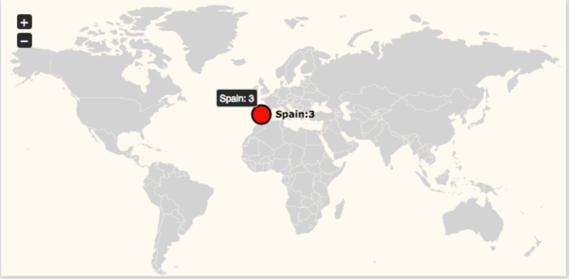