Federated Machine Learning (FML) for Financial Sector Threat Intelligence and Fraud Prevention
Machine learning has great potential to make decision-support processes smarter, cheaper, more automated, and self-improving. However, it is also recognized that widespread commercial adoption of machine learning will require approaches that offer sufficient guarantees about the security and privacy of the process of learning models, especially when companies wish to learn collaboratively from datasets that they locally maintain and control.
Indeed, there is an increasing need in the industry for sharing information and breaking data silos in order to really exploit multi-party or even cross-sectorial data and enable an open market of data-driven industries. It affects especially to a strict and highly-regulated sector such as the financial sector, in which data privacy and protection regulations are completely necessary but hindering those approaches. A solution that allows us to export data models and patterns from business data of one enterprise and make it available for others minimizing the exchange of sensitive data is a key component to be built. The usage of the customers’ data for security enhancement reasons is considered to be an exception in the regulation (e.g. GDPR article 23) but any exchange of real and raw data has a lot of concerns.
Therefore, financial institutions can benefit from Federated Machine Learning (FML) to build more accurate models in a collaborative way leveraging the datasets of different participants but preserving the privacy of the data. In other words, the participants in an FML task can benefit from the information provided by others to design a collaborative machine learning model, but this information is kept confidential for each participant.
In an FML task, there is a central node or server that is responsible for producing an aggregate machine learning model from the information sent by the different participants in the task. At training time, the server sends the participants the parameters of the shared model. The participants then compute locally, on their own datasets, and propose model updates that are sent back to the server. The information exchanged between the participants and the server could be updated versions of the parameters of the model, gradients, centroids, or other information relevant to update the collaborative machine learning model.
Among many of the applications that FML can have, in CONCORDIA we are exploring the application of FML in the financial sector especially for preventing cyberfraud. In the context of fraud detection, the advantage of such a collaborative machine learning model is clear: the participants can collaboratively obtain a better overall model that encompasses all the different types of fraud that each of the participants has detected, whilst preserving the privacy of their records. For example, a bank can have access to a model capable of detecting new types of fraud that have not been yet attempted against that bank, whilst other financial institutions may already have encountered them.
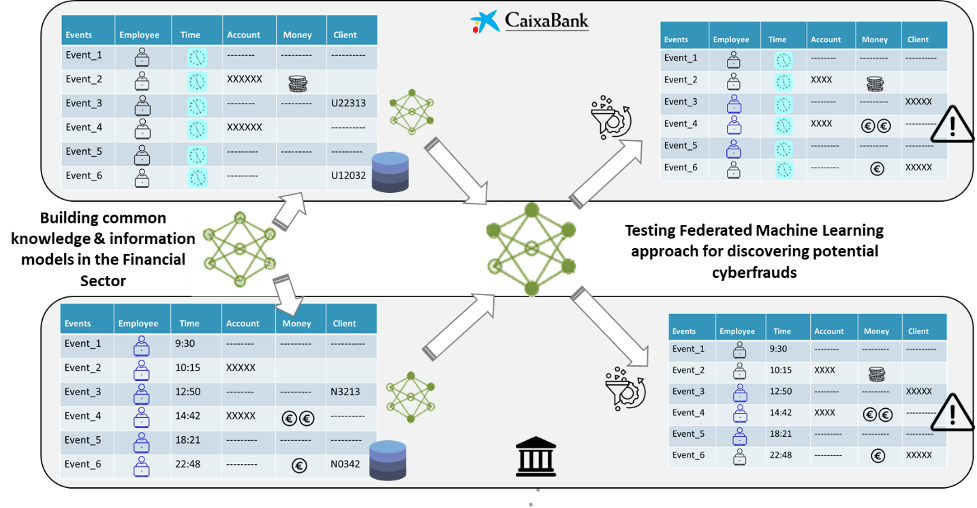
There are several challenges we are facing in the process. For example, in fraud detection we can expect datasets to be highly imbalanced, i.e. the fraction of fraudulent transactions is minimal compared to the number of legitimate ones. In this situation, training a standard supervised learning algorithm to detect fraud will catastrophically fail, as it will not pay attention to the tiny fractions of fraudulent transactions. This is a well-known problem in the research literature in machine learning. To solve the limitations of standard models we resorting to a set of techniques aiming to rebalance these skewed datasets or to give more importance to those data points that are a minority.
Another challenge is that fraud patterns change very dynamically and depend a lot on the fraud campaign and the fraudsters’ group. Therefore, supervised learning is not always effective to prevent future fraud and very little research has been done on the usage of FML for unsupervised learning. However, CONCORDIA pilot plans to explore both, unsupervised and supervised approaches to detect cyberfraud.
And last but not least, FML requires a consistent group of financial entities open to participate and collaborate on such an innovative approach. Therefore, CONCORDIA opened this pilot to other stakeholders outside the project and offered to collaborate to partners of other cybersecurity project pilots like CyberSec4Europe. Moreover, this is just the first step in the process of building a financial services community that collaborates much closer on cybersecurity and cyberfraud prevention.
Are you a financial entity willing to join us?
(By Ramon Martín de Pozuelo, CaixaBank)